Labor costs are the primary expense in the call center – research shows that agents and supervisors make up 70% of total call center expenses. This is a monumental cost, so it comes as no surprise that companies would want to make sure that their agents have all the necessary tools and training to ensure optimal agent performance.
In order to reach optimal agent performance, companies need to have a way of monitoring and measuring the KPIs that impact it. This is important because performance constantly evolves, and sometimes agents need "tune-ups" or reminders to maintain high-performance scores.
According to research conducted by SQM Group, a staggering 71% of senior call center leaders express a strong consensus that their primary objective is enhancing the customer experience (CX) within their call centers. Additionally, high-achieving call centers gauge CX success based on metrics such as First Call Resolution (FCR), Customer Satisfaction (Csat), and customer retention.
Additionally, SQM's research shows that 30% of calls are non-FCR for the call center industry. Agents are the source of error for 40% of non-FCR calls. Therefore, improving agent performance is a tremendous opportunity to enhance CX. AI can help companies accelerate this process by providing real-time actionable insights and feedback.
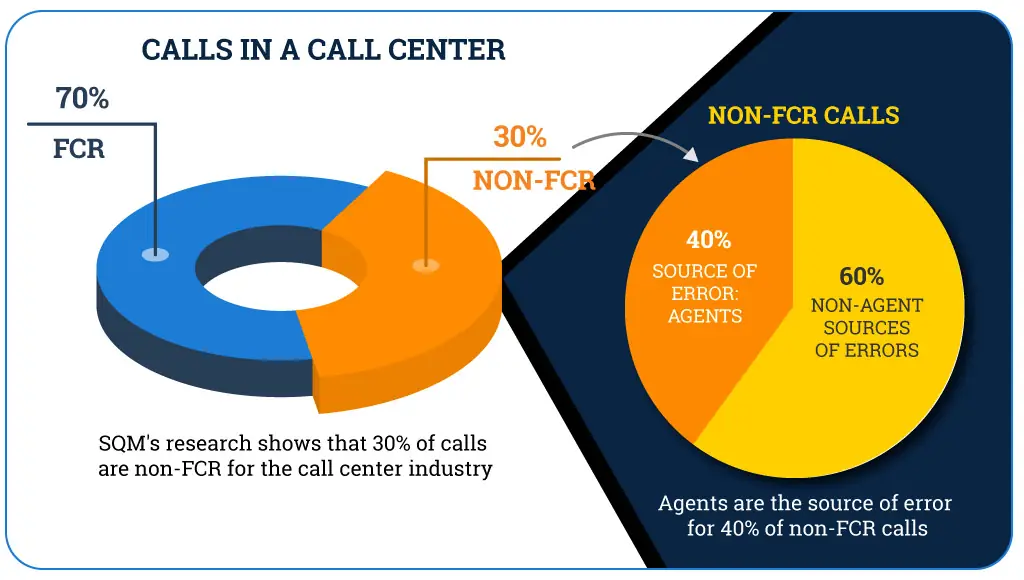
Instead of manually reviewing a sample of calls for quality assurance and agent performance evaluations, AI capabilities can review 100% of interaction data in real-time. This also provides agents with real-time call guidance. These AI technologies speed up the processes of measuring and improving agent performance and, as a result, reduce customer dissatisfaction and churn.
How Is Agent Performance Defined?
Call center agent performance refers to the effectiveness and efficiency of individuals working in a call center environment, primarily in delivering high-quality customer service and meeting predefined performance standards.
Agent performance encompasses a range of key performance indicators (KPIs) that evaluate an agent's ability to handle customer inquiries, resolve issues, maintain professionalism, adhere to call center scripts and guidelines, meet service level agreements, and achieve desired outcomes like customer satisfaction and retention.
Agent performance can be assessed through the following metrics:
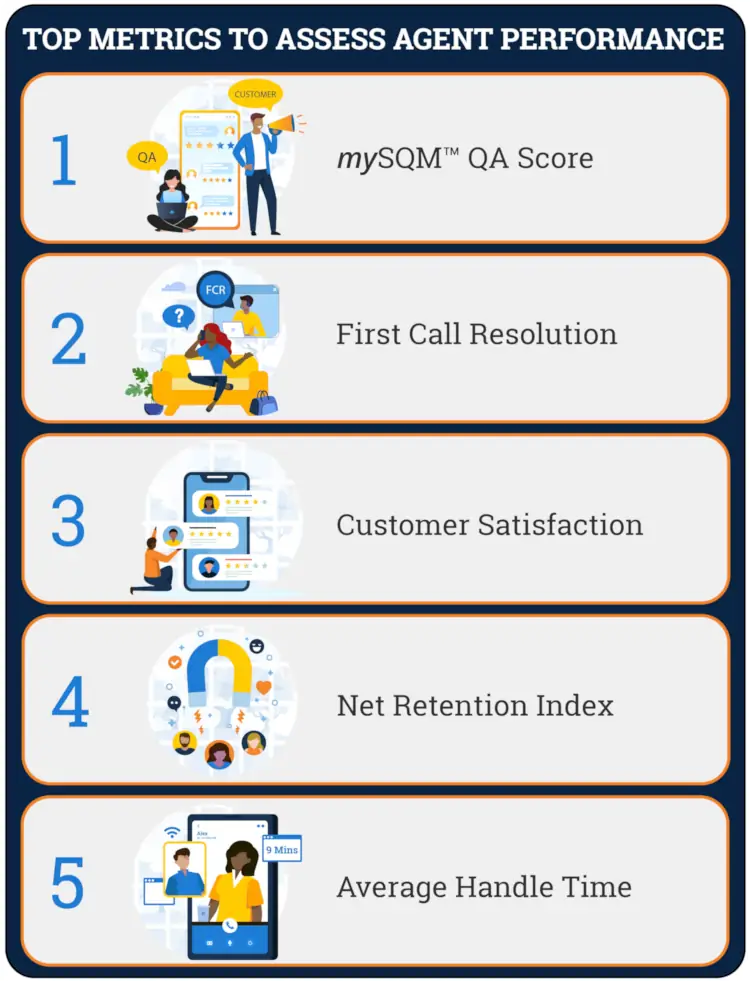
1. mySQM™ QA Score
To help call centers improve CX, we use a mySQM™ QA Score to measure agents, supervisors, and call center levels. The mySQM™ QA Score is comprised of customer experience and call compliance metrics. For the mySQM™ QA Score, 90% of the score is focused on CX, and 10% is focused on call compliance adherence. The mySQM™ QA Score uses AI technologies to capture, analyze, and report.
2. First Call Resolution
FCR measures an agent's ability to resolve a customer's issue during initial contact without requiring follow-up calls. A high FCR rate indicates that an agent efficiently solves problems and provides satisfactory solutions, a key indicator of agent performance.
3. Customer Satisfaction
Csat directly measures how satisfied customers are with their interactions with call center agents. Agents who consistently receive high Csat scores demonstrate their ability to meet customer needs, offer excellent service, and effectively communicate, showcasing strong performance.
4. Net Retention Index
NRI assesses the likelihood of a customer continuing to do business with the company after interacting with a call center agent. Agents positively impacting customer retention contribute to the company's long-term success, making this metric crucial for evaluating agent performance.
5. Average Handle Time
AHT evaluates the average time it takes for an agent to handle a customer call from start to finish. While lower AHT may imply efficiency, it should be balanced with maintaining quality service. Agents who strike this balance effectively showcase their proficiency in handling calls efficiently and ensuring customer satisfaction, making AHT a vital performance metric.
How Can AI Help to Measure and Improve Agent Performance?
Leveraging automated AI technologies such as call recording, speech analytics, natural language processing, automated scorecards, QA software, real-time monitoring, and data analytics in call centers can revolutionize how agent-customer interactions are monitored and analyzed in real time.
These AI-powered tools enable real-time capture and examination of a wide range of data from conversations, including tone, sentiment, call compliance, keywords, and even screen interactions. This data can then provide agents with instant contextual guidance during customer interactions.
By continuously analyzing conversations, AI can detect key indicators of customer sentiment and agent performance, such as signs of frustration, compliance with scripts, or deviations from best practices. Real-time contextual guidance based on these insights can help agents adapt their approach immediately.
For example, this guidance might include suggesting appropriate responses, offering alternative solutions, or notifying agents of relevant policies or information, all of which can lead to more effective and satisfying customer interactions.
Furthermore, the data collected and analyzed by AI technologies can be used to measure and improve agent performance in the following three ways:
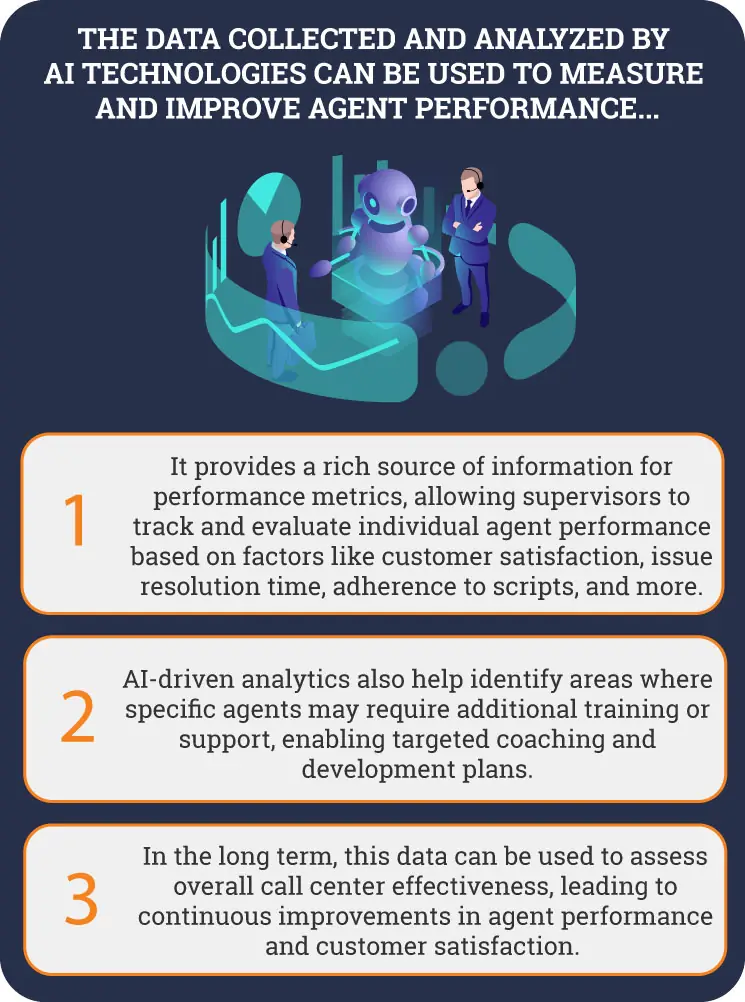
More specifically, AI can help call centers by measuring and providing guidance on the top five metrics used to assess agent performance:
SQM Group has a proven track record for helping call centers improve customer service QA. To help call centers improve CX, we use a mySQM™ QA Score, which is a standardized approach, to measure agents, supervisors, and call center performance. The mySQM™ QA Score is tracked and can be used to benchmark agent, supervisor, and call center performance within an organization and against other organization call centers.
SQM’s research shows that automating QA has had success in measuring and improving call compliance but has had moderate to low success in improving CX. The mySQM™ QA Score focuses on helping call centers improve CX by using AI technologies and the right metrics.
AI-based quality assurance tools can continuously assess agent-customer interactions for delivering a great CX and adherence to predefined quality standards. If deviations are detected, AI can provide guidance and coaching recommendations to ensure agents align with established quality benchmarks.
However, there are some design issues in the QA programs that many call centers use that need to be addressed and fixed before QA programs can be automated. For example, most agents are evaluated on 4 to 10 calls per agent per month, which is a small sample size according to most agents and supervisors. Furthermore, QA evaluators can be inconsistent with their QA evaluation scoring, inefficient for evaluating calls, and expensive.
It is also common for QA programs to use the wrong metrics that have little or no impact on Csat. For example, it is common for QA evaluators or Artificial Intelligence (AI) evaluations to measure if the agent used the customer's name multiple times during the call to make the customer feel the interaction was personalized and thus assumed it improved Csat. However, research shows that this call-handling technique has very little or no impact on Csat.
Auto-QA and compliance processes eliminate or significantly reduce manual QA evaluations by automating them. By scoring 100% of calls with AI, you can remove human errors and agent biases from the call center QA program. Auto-QA and compliance is a more effective way to manage QA and compliance because it dramatically increases the scale of QA evaluations by going from 1 to 2% up to 100%. Therefore, you can identify compliance issues in real time to take the necessary action.
However, it's important to note that while AI can significantly enhance call center QA and compliance processes, it may not completely replace the need for human involvement. Human QA experts are still essential for nuanced evaluations, making judgment calls, and providing context that AI may not fully grasp. A combined approach, where AI assists human QA teams, often yields the best results in terms of both efficiency and accuracy.
AI can also be used to improve agent performance through agent self-coaching. Agent self-coaching uses AI-generated personalized coaching suggestion CX best practices to help agents improve their performance.
The Agent Self-Coaching component in mySQM™ Customer Service QA Software has three key features:
1. Customer Feedback to Improve CX
SQM uses conversational IVR/IVA surveys to turn qualitative feedback from customers into actionable coaching outcomes. In other words, to coach agents effectively, you need an explanation of why customers are dis/satisfied or why their call was not resolved to understand how to improve CX.
2. Coaching Opportunity Identification
Agent Self-Coaching uses an agent dashboard and QA scores to identify coaching opportunities to improve performance.
3. AI-Generated Personalized Coaching
Agent Self-Coaching uses AI-generated personalized coaching suggestions to improve their Csat and QA performance.
Request a mySQM™ Customer Service QA Demo Video
Learn about SQM's agent… Csat prediction model, which is based on standardized metrics, AI, and regression analysis to predict customer satisfaction derived from a QA evaluation. Our Csat prediction model provides a high statistical correlation, translating to a 95% success rate in predicting agent Csat in most cases.
Speech and text analytics can monitor real-time interactions to determine whether customer inquiries are resolved during the initial call. By identifying missed opportunities for FCR, the analytics system can provide agents with real-time guidance on problem-solving, thereby increasing the likelihood of resolving issues during the first interaction.
Conversational IVR survey methods are another way to detect whether FCR has been achieved. Conversational IVR surveys can ask customers questions about whether their call was resolved. The conversational IVR method is designed to simulate a human conversation to conduct a post-call survey over the phone. Instead of using a static and rigid menu of options, the conversational IVR survey can understand and respond to the respondent's answers, allowing for a more dynamic and personalized survey experience. For example, based on a respondent's previous answer, the conversational IVR survey will follow up with a relevant question.
By tracking FCR rates over time, companies can identify patterns in areas with lower FCR and take corrective actions. This improves CX and increases agent efficiency and effectiveness.
AI can analyze customer feedback and sentiment during conversations to provide immediate insights into customer satisfaction. If AI detects signs of dissatisfaction or frustration, it can offer agents real-time suggestions for improving the customer experience, such as empathy and de-escalation techniques.
AI algorithms can use time-related analysis, interaction duration, and agent performance metrics to predict Csat scores. By forecasting Csat scores for specific interactions, companies can pinpoint potential concerns and proactively enhance customer satisfaction.
A great example is the ability to measure Csat through Conversational IVR/IVA survey methods. This conversational IVR survey imitates a human voice to simulate human conversation. Through conversational IVR/IVA survey methods, speech analysis, and sentiment analysis, the conversational IVR survey can capture post-call customer feedback to provide valuable insights into the call center's Csat, FCR, QA, and NPS performance and identify improvement opportunities.
AI can track customer behavior and engagement post-interaction to evaluate the impact of the call on customer retention. It can identify areas where agents can contribute to better retention and provide guidance accordingly, such as upselling opportunities or strategies to enhance customer loyalty.
With call centers typically being used as the last line of defense, customer service over the phone is more important now than ever before. Therefore, maximizing customer retention by leveraging the analytics and guidance that AI technology is capable of is crucial.
Customers rely on call centers to resolve issues or inquiries, especially when other means of communication, such as email or live chat, do not suffice. This means that excellent customer service in the call center is essential for high customer retention.
SQM Group's research shows that the number one thing customers expect from call centers is fast resolution for resolving their inquiries or problems. Therefore, Average Handle Time (AHT) is essential to monitor because it directly relates to a fast resolution for resolving customers' inquiries or problems.
AI analytics can monitor AHT and suggest ways for agents to streamline their interactions without compromising service quality. For instance, AI can identify time-consuming processes that can be automated or offer tips on improving efficiency during calls.
AI can also be integrated to automate routine tasks and optimize call routing. For example, AI-powered chatbots and conversational IVR survey can handle simple inquiries, reducing agent workload and AHT.